If you are a marketer who relies on AB testing to capture insights about your target market and make your advertising campaigns more cost-efficient, you can benefit from knowing and applying the time-tested lessons of scientific experimentation.
As pioneers of the high-tempo testing approach, we would like to believe that everything we do is original. However, by studying the history of AB testing, we developed a deeper appreciation for the true AB testing pioneers and have been able to apply their knowledge to what we do in 2020.
To create this article, we combed through the Woodridge Growth library—which includes books, academic white-papers and journal articles—and surfaced the most relevant lessons for modern marketers.
To be clear, this post is not meant to be a history lesson. If it was, we would refer back 300 years ago to, when James Lind was searching for the cause and cure for scurvy (see: James Lind, “A Treatise of the Scurvy” from 1753).
AB Testing Lesson #1: Multiple Working Hypotheses
Begin your tests with multiple hypotheses—rather than just one—so that your “intellectual affections” for a certain theory don’t obstruct the truth.
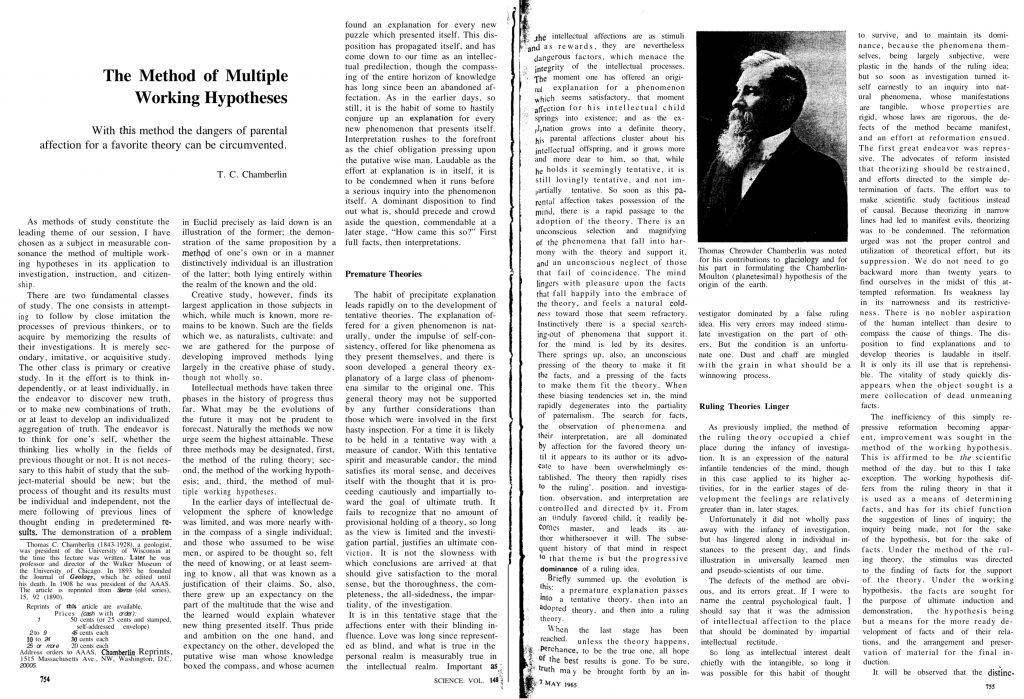
The 19th-century American geologist T.C. Chamberlin is known for introducing a method central for avoiding bias at one of the earliest stages of all scientific experiments: the hypothesis.
In 1890 Chamberlin published a paper titled “The Method of Multiple Working Hypotheses” that challenged the long-established norm in scientific research of testing one primary hypothesis.
Chamberlin argued that testing one hypothesis before formulating alternatives creates barriers for the pursuit of scientific truth:
- Natural phenomena tend to be more complex than can be explained by a single hypothesis.
- If we work with only one hypothesis, it’s likely that we’ll (consciously or unconsciously) seek out evidence that supports that hypothesis, rather than leave open the idea that different—or multiple—theories could provide a more rational explanation. Chamberlain called this bias a problem of intellectual affection.
Chamberlin writes extensively on the second problem in his paper:
It is the habit of some to hastily conjure up an explanation for every new phenomenon that presents itself. Interpretation rushes to the forefront as the chief obligation pressing upon the putative wise man. Laudable as the effort at explanation is in itself, it is to be condemned when it runs before a serious inquiry into the phenomenon itself. A dominant disposition to find out what is, should precede and crowd aside the question, commendable at a later stage, “How came this so?” First full facts, then interpretations.
The Method of Multiple Working Hypotheses
As a solution, Chamberlin proposed his method of multiple hypotheses, which has us consider from the outset many different hypotheses to explain a given phenomenon, reject those that conflict with our data, and do so iteratively until we are left only with the theories that fit our results best.
Although AB tests differ in methodology from the geological research Chamberlin performed, the method of working hypotheses remains a reliable way to mitigate your biases in testing.
AB Testing Lesson #2: The Null Hypothesis
Assume from the outset that there is no relationship between your two variables, and consider your AB tests as an effort to show explicitly that there is one.
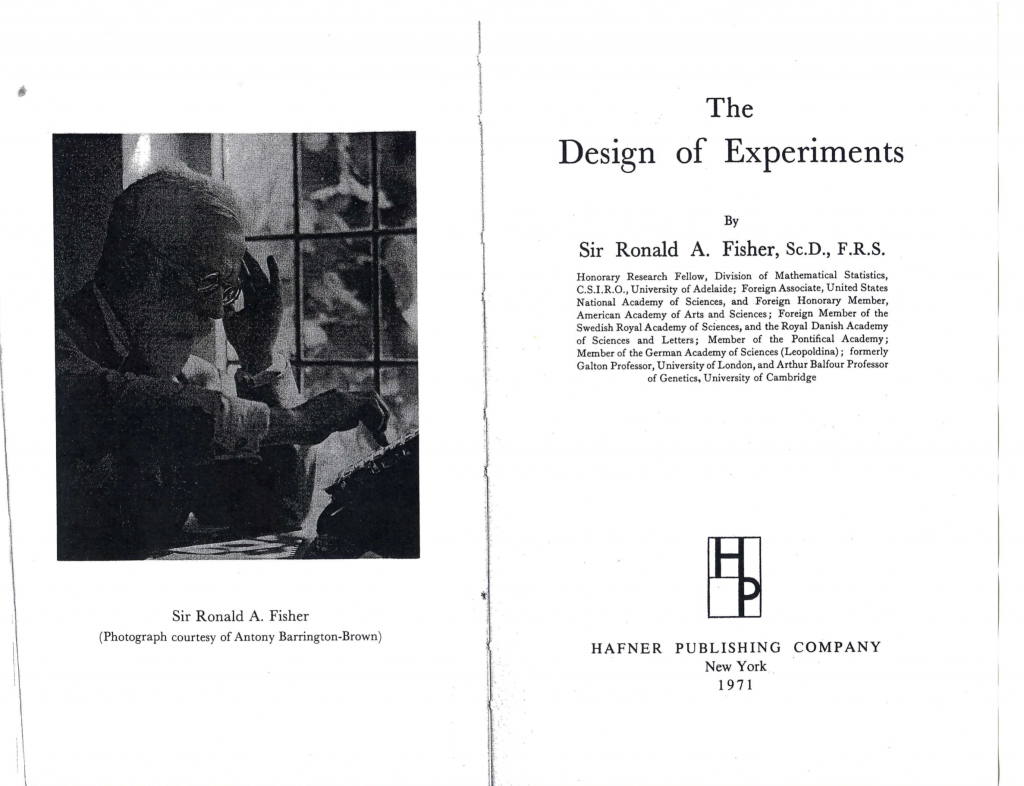
Several decades after Chamberlin published “The Method of Multiple Working Hypotheses,” the British statistician Ronald Fisher began devising the fundamentals of AB tests.
Kaiser Fung, the author of Junk Charts and numerous books on data science, emphasizes the significance of Ronald Fisher’s contributions to what we know as AB testing today:
In the 1920s statistician and biologist Ronald Fisher discovered the most important principles behind AB testing and randomized controlled experiments in general. “He wasn’t the first to run an experiment like this, but he was the first to figure out the basic principles and mathematics and make them a science,” Fung says.
Harvard Business Review, A Refresher on AB Testing
What led Ronald Fisher to pioneer these methods? In 1919, Fisher was hired by the Rothamsted Experimental Station to analyze the data they had collected comparing the effect of fertilizers on various crops.
It was here that Fisher began to develop the robust statistical methods he introduced in his book The Design of Experiments (1935).
One of the principles Fisher introduced in The Design of Experiments is the null hypothesis. The null hypothesis is the universal assumption that there is no relation between any two variables—whether they’re fertilizers and crop growth, or call to action text and click-through rate.
Fisher demonstrated that if a test is to prove that the apparent effect of an independent variable on a dependent variable is a result of anything but sheer randomness (i.e., statistical noise), the results must demonstrably reject the null hypothesis, which is held to be true until proven otherwise.
Rejecting the null hypothesis depends on the statistical significance of a test’s results, or the probability that the results would appear even if the null hypothesis is true and the independent variable has no effect on the dependent variable. i.e., it’s the probability that the results are due only to randomness.
AB Testing Lesson #3: The Value of Small-Scale Tests
Scientific tests have had demonstrable use to advertisers for nearly a century. Test on a small scale and be conscious of your budget: pursue the goal of advertisements that are maximally cost-effective, i.e., profitable.

Claude Hopkins, a pioneer of direct-response advertising, brought scientific thinking to a non-laboratory environment and proved the quantitative value of advertising to business executives across the country.
In his 1923 book Scientific Advertising, Claude Hopkins introduced the concept of test campaigns, a cost-effective method for designing and uncovering the highest-possible-performing advertisements.
One of Hopkins’ central messages throughout Scientific Advertising is that advertisements should be designed with one goal in mind: maximizing profits.
The only purpose of advertising is to make sales. It is profitable or unprofitable according to its actual sales.
Ads are not written to entertain. When they do, those entertainment seekers are little likely to be the people whom you want.
Scientific Advertising
Hopkins conceived of test campaigns as an approach to finding the most profitable advertisements—the ads that yield the most efficient cost-per-sale—without breaking the bank.
Test campaigns consisted of different versions of an ad for a certain product that Hopkins mailed to various cities. Hopkins would measure the sales and cost-per-sale of each version with precision and proceed to use the highest-performing ad in a national campaign.
Now we let the thousands decide what the millions will do. We make a small venture, and watch cost and result. When we learn what a thousand customers cost, we know almost exactly what a million will cost. When we learn what they buy, we know what a million will buy… We know our cost, we know our scale, we know our profit and loss… Before we spread out, we prove our undertaking absolutely safe.
Scientific Advertising
Learn what works with a small amount, then spend aggressively behind what you know drives profitable sales.
AB Testing Lesson #4: Know Your Environment
When you run an AB test on Facebook, you’re not testing in an airtight controlled environment like Fisher, or even the relatively stable mail-order domain of Hopkins. Facebook’s complex, interconnected social networking environment has repercussions for your tests.
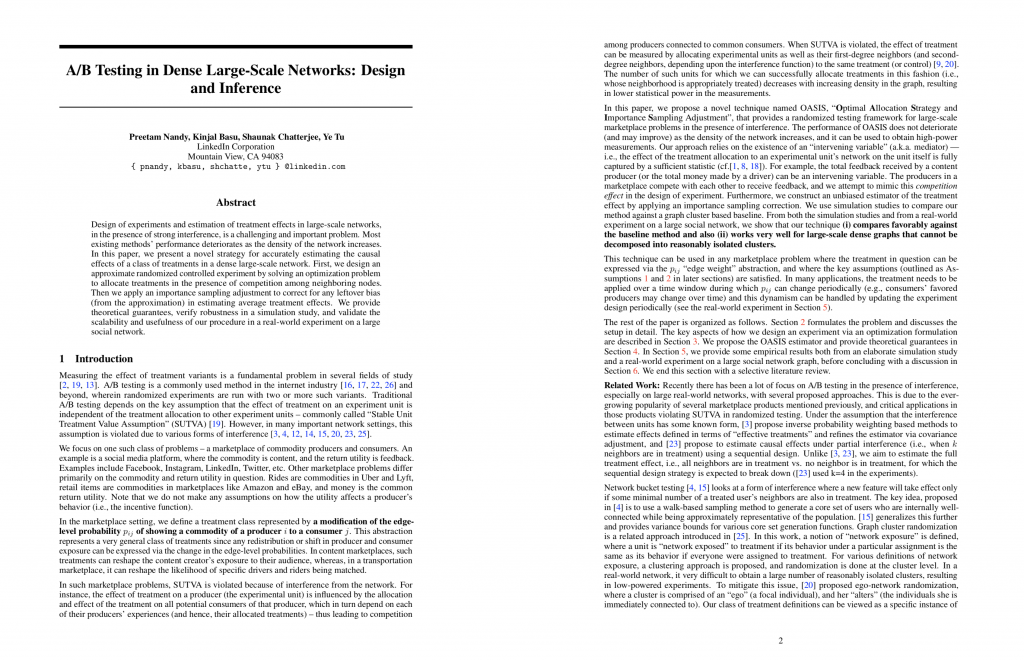
It’s important to be aware of the limitations imposed on us by the nature of the environment we perform tests in.
Statisticians like Ronald Fisher had the luxury of analyzing experiments performed in laboratory settings where the environment is controlled and all of the variants being tested are designed to be completely independent of each other.
Even Hopkins, whose mail-order environment was far from as controlled as that of a scientist like Fisher, could consider the ad performance in a one city as relatively unlikely to be affected by the performance of a different ad in a city across the country.
Traditional AB tests, like those Fisher researched, are considered statistically valid thanks to the Stable Unit Treatment Value Assumption (SUTVA).
Fisher knew that if fertilizer was given to Crop A, it had no impact on the growth of Crop B, because Crop A and Crop B exist completely independent of one another.
The problem is this: SUTVA doesn’t hold true for AB tests performed on Facebook.
A paper by LinkedIn engineers published in 2019 titled “AB Testing in Dense Large-Scale Networks: Design and Inference” discusses the problems with running truly scientific AB tests on complex, large-scale social networks.
Facebook is an environment in which the users are neither independent or controlled. Furthermore, the interactions you have with people off of Facebook impact what you (and/or they) will see on their Facebook News Feed. We’ll go more into detail on this topic in a future post, but in short, the nature of online social network systems make it impossible to ensure SUTVA holds.
Unless changes are made to Facebook’s split-testing algorithms that specifically account for this violation of SUTVA, the possibility of interference between tests is essentially unavoidable. And even if SUTVA is not violated, we cannot prove it…
It’s not a perfect environment, which is why we advocate acting on test results that show big differences vs. the control.
Conclusion
It is our belief that in order to create the future of marketing, we must have a firm appreciation for the past. In marketing, and in life, there are things that change often and things that change slowly or not-at-all.
In ten years, we may not use Facebook to reach our target market. The channels will change, and change often. There is another bucket-of-knowledge that is characterized by slow-to-no change. This is what is worth studying, mastering, and applying to the innovative marketing work you do. We hope this article helps.
Leave a Reply